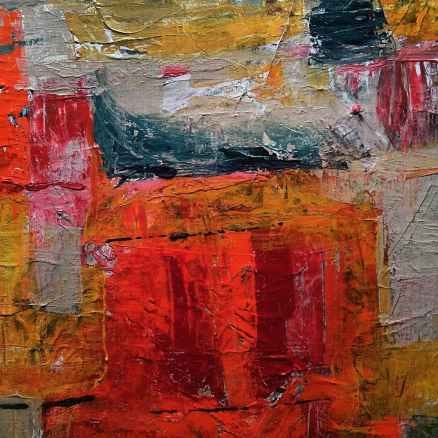
The science of complex adaptive systems is a biological model of systems change. It has a scientific method as robust as the mechanical method we were taught in school, but accounts for the prevalence of unpredictability in how the real world operates.
Economic models tend to be wildly over simplistic. The standard of introductory econ courses is to teach how two variables interact with each other while holding all else constant. Then as the student progresses we add these variables on top of each other segment by segment to build an example of how a complete economic system might work. In her book Econned Economist Yves Smith gives multiple examples of how this cumulative effect in economics fails to accurately address economic problems.
demand function fails as a ‘scientific’ theory when extended from a single consumer to multiple consumers… [using] the construct of a single consumer whose tastes do not change with income or over time, as a proxy for the society-wide demand function.
A simple exercise in grocery shopping in the era of COVID illustrates the problem of a demand curve that operates solely in regard to volume and price. When we put together our shopping list for curbside pick-up we are given a variety of choices in the app if they do not have what we requested. The default choice is best available. This does not allow for the fickle nature of human taste, however. If they do not have frozen broccoli florets is the next best item a simple frozen broccoli, is it fresh broccoli, is next best even a vegetable? If I plan on making a frittata and can’t get the broccoli maybe I don’t want more eggs either and I want marinara and pasta instead. An easy switch to make in the store, impossible in a shopping app.
Adding income into the mix we can see the basic supply demand curve model move even further from real world choices.
Our prototypical consumer, who was as satisfied with eight [apples] and one [bunch of bananas] as he was with six [bunches of bananas] and two [apples], is assumed, at a higher level of income, to be as happy with eighty [apples] and ten [bunches of bananas] as with sixty [bunches of bananas] and twenty [apples].
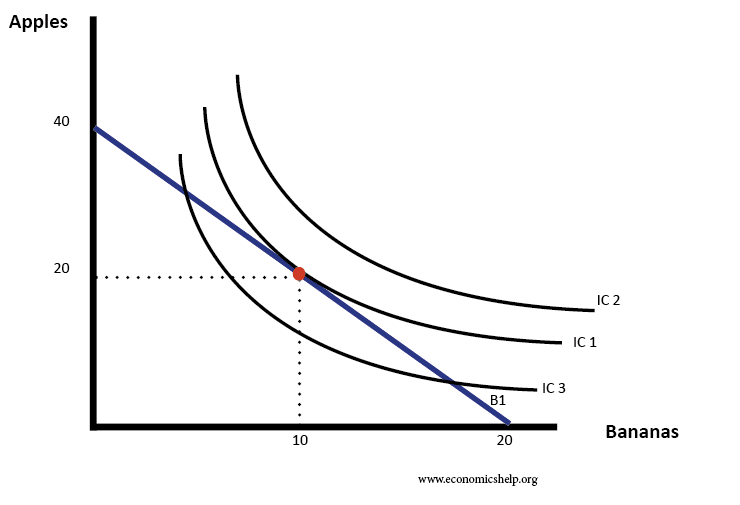
So how did we get here, where oversimplification in service of making the models work takes us further and further from the way we observe the economy working in real time? The answer can be found in eighteenth century political economy. The age of enlightenment was a time where great strides were made in western society’s understanding of how the world worked. These intellectual discoveries were largely driven by discoveries in mathematics that were applied across disciplines. Algebra, calculus, and geometry opened windows on how we were able to perceive the world around us. These maths were quickly applied to problems of economic theory.
As these disciplines grew they also became siloed. Physics and economics became separate fields in separate disciplines and each went their merry way. The sciences of psychology and biology also developed. While mechanistic models (same input always leading to the same output—test—rinse—repeat) still answered some scientific questions it was insufficient for others.
Then in 1982 Santa Fe happened. A number of scientists from multiple disciplines: physics, biology, psychology, computer science, economics were brought together to develop a cross disciplinary approach to their fields. This cross disciplinary approach was based on advances in the study of complex adaptive systems, a biological model of change. Where a mechanistic method was insufficient to explain real world phenomena, the biological model filled in the gaps. There are some common characteristics of complex adaptive systems—emergence, field dependency, and feedback loops among them which explain real world phenomena in ways that account for the interactions of variables. Science was no longer a zero sum game. Economics had some catching up to do.
The value of using a biological model to understand change is that it gives us a method for predicting the unpredictable and for influencing tipping points with intention.
Subscribe at beth.substack.com